Movie Title
Noisedive
Artist/Director
Year
2015
Country
USA
Added
Resolution
720
Description
This is what emerges when you recursively feed noise to a artificial neural network trained to recognise places (e.g. you give it an image and the response is something like 90% beach , 20% desert, 2% swimming pool). The familiar shapes you see floating past is abstractions the network has made for the different categories. Some categories feature more prominently because the amount of images used to train them differ. For example the fountain category contains 111,496 images compared to only 883 in the nuclear_power_plant one. So that s why you see a lot more fountain like shapes than big chimneys. There s a lot more to it than that of course, and this visualisation only shows you one of the many abstraction layers the network uses. Here s some of my favourite places from this dive: johan-nordberg.com/deepdreams/noisedive1/blueberry_falls.png johan-nordberg.com/deepdreams/noisedive1/bubblegum_land.png johan-nordberg.com/deepdreams/noisedive1/great_gatsby.png johan-nordberg.com/deepdreams/noisedive1/iceberg_caves.png Rendered on Amazon EC2 using their g2.2xlarge GPU instance, total render time was 38 hours. Based on the work by Google researches Alexander Mordvintsev, Christopher Olah and Mike Tyka googleresearch.blogspot.com/2015/06/inceptionism-going-deeper-into-neural.html googleresearch.blogspot.com/2015/07/deepdream-code-example-for-visualizing.html The places image database including a selection of pre-trained neural networks can be found on places.csail.mit.edu
Movie Image
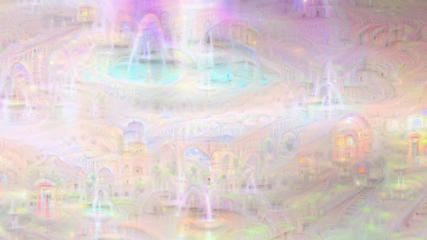
Duration
0:04:52